NIST Sets Standards for Differentially Private Data Protection
NIST's new guidelines build upon their 2017 publication, "Differential Privacy: A Primer for a Digital Age."
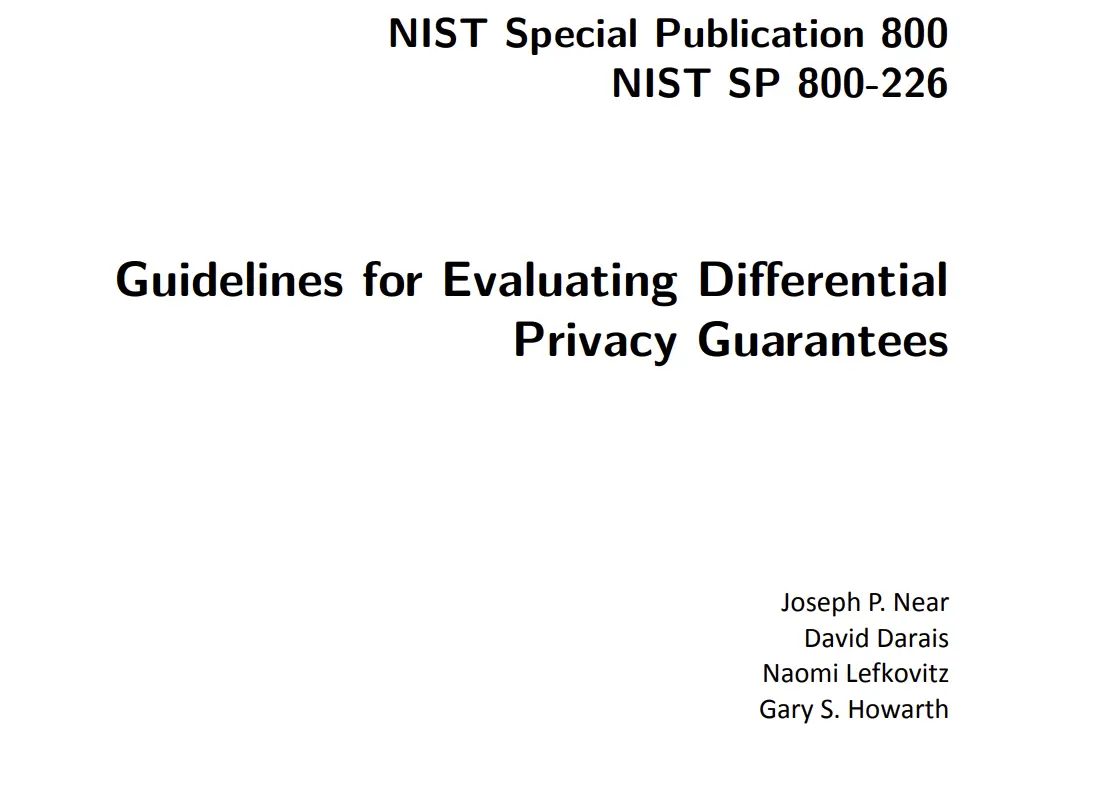
The National Institute of Standards and Technology (NIST) has recently released updated guidelines on differential privacy, a framework designed to protect personally identifiable information when sharing data. This move comes as organizations face increasing pressure to balance the need for data-driven decision-making with the responsibility to safeguard individuals' privacy.
Differential privacy is a mathematical technique that introduces carefully calibrated noise into datasets to prevent the identification of specific individuals while still allowing for useful analysis. The concept has gained traction in recent years as a potential solution to the privacy dilemmas posed by big data and artificial intelligence. However, some experts remain cautious about its real-world applications and potential limitations. NIST's new guidelines build upon their 2017 publication, "Differential Privacy: A Primer for a Digital Age."
The updated document provides more detailed recommendations for organizations seeking to implement differential privacy in their data-sharing practices. It includes sections on risk management, transparency, and user choice, as well as best practices for designing and deploying differentially private systems. The institute's efforts to provide clear guidance on differential privacy are commendable, especially given the complexity of the subject matter. However, some concerns persist about the feasibility and effectiveness of this approach in various contexts.
For instance, while differential privacy can offer robust privacy guarantees in theory, its practical implementation may not always align with these ideals. Factors such as data quality, noise levels, and the specific algorithms used can all impact the actual privacy protections afforded by differentially private systems. Moreover, the reliance on mathematical models to ensure privacy raises questions about accountability and oversight.
If a differentially private system fails to provide adequate protection, it may be unclear who should bear responsibility—the organization that implemented it, the developers who created the algorithms, or the researchers who designed the noise-addition mechanisms. It is also worth noting that differential privacy is not a one-size-fits-all solution.
Organizations must carefully consider their unique data-sharing needs and privacy concerns when deciding whether to adopt this approach. In some cases, alternative methods—such as data anonymization or secure multi-party computation—may offer more suitable solutions for balancing data utility and individual privacy. In conclusion, NIST's updated guidelines on differential privacy represent a significant step forward in promoting responsible data sharing practices. However, organizations should approach this technique with caution and recognize its limitations. As with any emerging technology, a healthy dose of skepticism is essential to ensure that the promises of differential privacy are realized without sacrificing individual privacy or data security.